Customer Churn Prediction: Identify and Optimize!
Reading Time: 10 minutes
Predicting customer churn for businesses is akin to forecasting bear markets for investors. While the exact timing is uncertain, churn is inevitable. The dread of churn is universal, but it’s crucial to acknowledge that a certain percentage of customers will inevitably part ways. Unlike the unpredictable nature of bear markets, customer churn can be anticipated and mitigated with precision.
With the right data, tools, and strategies, businesses can forecast churn and implement measures to prevent it, thereby lowering their churn rates and sustaining higher revenue streams. Predictive analytics and strategic interventions make it possible to foresee which customers are at risk and take proactive steps to retain them.
In this blog post, we will discuss the phenomenon of customer churn, exploring its causes, critical warning signs, and methodologies to predict and minimize its impact. By understanding and addressing these elements, you can significantly enhance customer retention and safeguard your business’s financial health.
What is Churn?
Churn occurs when a customer ceases to use your product or service, effectively ending their relationship with your business. This can happen for various reasons, from dissatisfaction with the product to finding a better alternative. The churn rate is a critical metric that quantifies this phenomenon, representing the percentage of customers who discontinue their engagement with your offerings over a specified period.
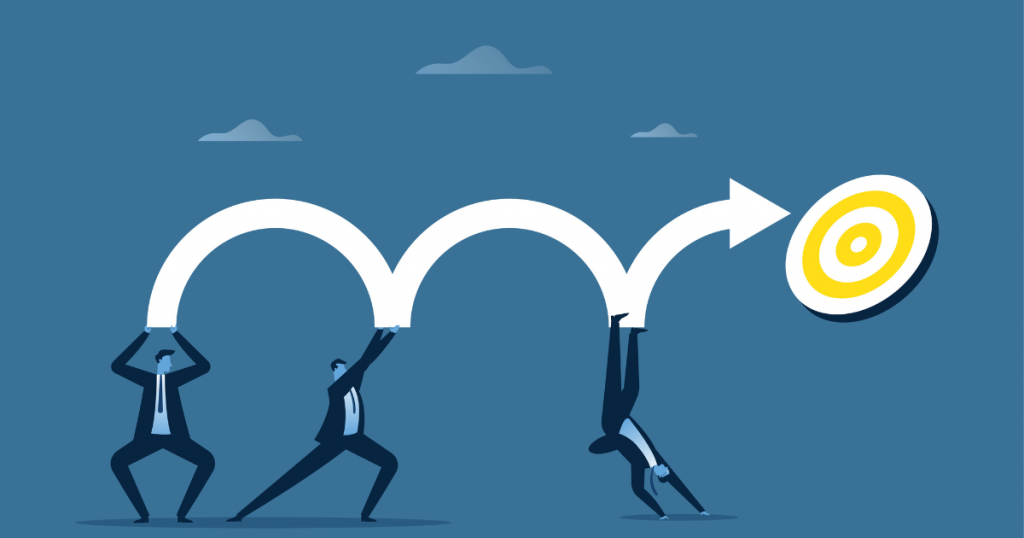
Understanding churn is vital for fostering long-term customer relationships and maintaining steady revenue growth. By closely monitoring and analyzing your churn rate, you can gain valuable insights into customer behavior and preferences.
This enables you to identify patterns and trends, uncover root causes of dissatisfaction, and implement targeted strategies to enhance customer retention. Effectively managing churn is about understanding your customers’ needs and continuously improving your offerings to meet those needs more effectively.
How is Churn Calculated?
Calculating the churn rate is a straightforward yet essential process for understanding the health of your customer base. The formula for churn rate is:

This calculation provides a percentage indicating the proportion of customers who have stopped using your product or service during a specific period.
To break it down further:
- Lost Customers: This is the number of customers who have discontinued their use of your product or service within the given time frame. Accurate tracking is crucial for precise calculations.
- Total Customers at the Start of the Time Period: This is the number of active customers at the beginning of the period you are analyzing. This baseline figure contextualizes the number of lost customers, helping to gauge the severity of the churn.
For example, if you start with 1,000 customers at the beginning of the month and lose 50 by the end of the month, your churn rate would be:

Understanding your churn rate is the first step in developing predictive models that forecast future churn, enabling you to proactively address potential problems and reduce customer attrition.
While customer churn measures the number of customers who stop using your product or service over a specific period, revenue churn focuses on the financial impact of lost customers. Revenue churn measures the amount of recurring revenue lost when customers stop using your product or service or downgrade their service levels. The formula for revenue churn is:

For example, if your total revenue at the beginning of the month was $100,000 and you lost $5,000 in revenue due to customer cancellations or downgrades, your revenue churn rate would be:

In summary, customer churn measures the number of lost customers, while revenue churn measures the financial impact of those lost customers or downgraded services. Both metrics are crucial for understanding different dimensions of churn and developing comprehensive strategies to retain customers and stabilize revenue.
What is Customer Churn Analysis?
Customer churn analysis involves meticulously examining your current churn rate, understanding the triggers that cause customers to leave, and identifying the behavioral traits they exhibit before churning. This analytical approach not only helps you comprehend the underlying causes of churn but also equips you with the insights needed to predict and mitigate it more effectively.
Key Aspects of Churn Analysis:
- Understanding Churn Triggers: By identifying what prompts customers to churn, you can gain a deeper understanding of their motivations and pain points. These triggers could range from dissatisfaction with your product or service to more attractive offers from competitors, poor customer service experiences, or unmet expectations. Recognizing these triggers allows you to address them directly, thereby improving customer satisfaction and loyalty.
- Analyzing Behavioral Traits: Customers often exhibit specific behaviors before they churn, such as a decline in product usage, increased complaints, longer response times, or engagement with competitors. By tracking these behavioral indicators, you can identify at-risk customers early and intervene before they decide to leave.
- Monitoring Churn Rate: Keeping a close eye on your churn rate over time helps you identify trends and patterns. Understanding when and why spikes in churn occur can reveal valuable insights into the factors driving customer attrition.
Benefits of Churn Analysis:
- Identifying Weak Points in Your Product or Service: Churn analysis can reveal areas where your product or service may not be meeting customer expectations. Addressing these weak points can help reduce churn.
- Enhancing Customer Engagement and Communication: Effective engagement and communication are critical for retaining customers. Churn analysis can highlight opportunities to improve how you interact with your customers, whether through personalized communication, more responsive support, or targeted marketing efforts.
- Predicting and Preventing Churn: One of the most powerful outcomes of churn analysis is the ability to predict which customers are likely to churn. By developing predictive models based on historical data and identified churn triggers, you can proactively reach out to at-risk customers with tailored interventions, such as special offers, personalized support, or loyalty programs designed to re-engage and retain them.
Now, let’s get into customer churn prediction.
What is Customer Churn Prediction?
Customer churn prediction is a system that businesses use to anticipate churn before it happens. This allows them to take proactive steps such as offering discounts, resolving issues, or re-engaging customers to prevent churn. Retaining existing customers is often much more cost-effective than acquiring new ones, which is why monitoring customer churn prediction and maintaining low churn rates are critical for businesses.
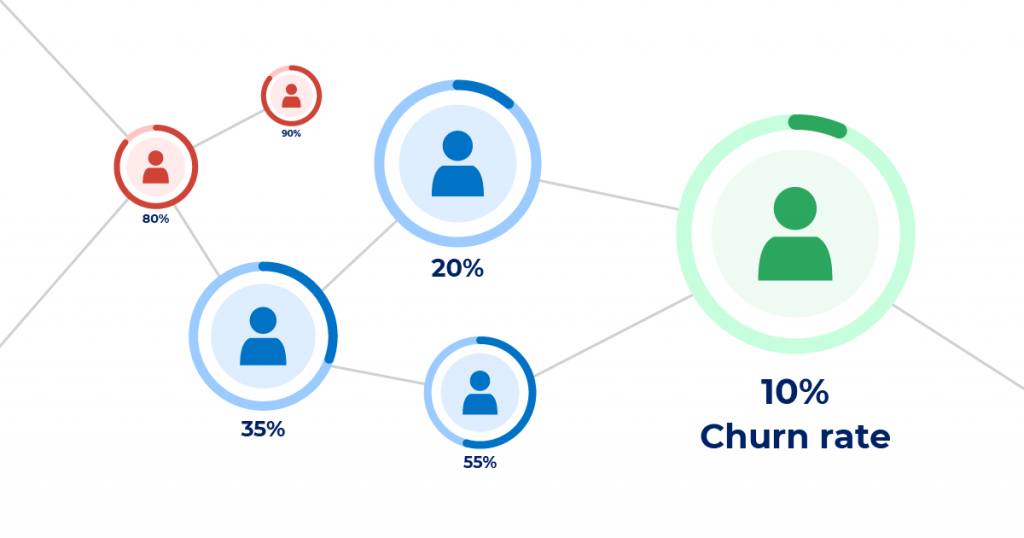
Exploring the Churn Prediction Model
A churn prediction model uses data from churn analysis to identify customers likely to churn based on their behavioral traits. These traits might include infrequent product or service usage, not deriving value from the service, having unresolved grievances, submitting support tickets, not renewing, and recent reductions in activity.
To build an effective churn prediction model, several key elements must be meticulously implemented. These elements include data collection, segmentation, and data analysis.
1. Data Collection
The foundation of any churn prediction model lies in robust data collection. This involves gathering comprehensive data on customer interactions with your product or service. For SaaS products or other online services, this means capturing user engagement and behavioral data. Key data points to collect include:
- Usage Frequency: Track how often customers use your product or service.
- Feature Utilization: Monitor which features are used most frequently and which are neglected.
- Customer Feedback: Collect feedback through surveys, reviews, and support tickets to gauge customer satisfaction and pain points.
- Support Interactions: Record the number and nature of support requests to identify recurring issues.
- Transactional Data: Log purchase histories, renewal rates, and subscription statuses.
This data provides a holistic view of customer behavior, enabling the identification of patterns that precede churn.
2. Segmentation
Segmentation is the process of dividing your customer base into distinct groups based on various criteria. By analyzing historical data, you can create segments that reflect different customer profiles and their associated churn risks. Common segmentation criteria include:
- Contract Types: Different contract terms (monthly, annual, etc.) can influence churn behavior.
- Revenue Information: High-value customers may have different churn predictors compared to low-value customers.
- Demographics: Age, location, industry, and other demographic factors can impact customer retention.
- Behavioral Segments: Group customers based on their engagement levels, feature usage, and interaction patterns.
Segmenting your customer base allows for more precise predictions and tailored retention strategies. For example, high-value customers might receive more personalized attention, while customers on monthly contracts might be targeted with incentives to switch to annual plans.
3. Data Analysis
Data analysis is the core of churn prediction. Once you have collected and segmented the necessary data, the next step is to analyze it to identify customers who are at risk of churning. This can be done manually or, more effectively, through automated tools and machine learning algorithms.
- Manual Assessment: Reviewing data to spot trends and patterns. This approach can be labor-intensive and may not scale well.
- Automated Tools: Leveraging software to process large datasets quickly. These tools can identify complex patterns and correlations that may not be immediately obvious.
- Machine Learning Models: Utilizing algorithms to predict churn based on historical data. These models can continuously learn and improve their accuracy over time. Common techniques include logistic regression, decision trees, and neural networks.
The analysis should focus on identifying key predictors of churn, such as reduced usage frequency, increased support requests, and negative feedback. By pinpointing these indicators, you can create a risk profile for each customer.
4. Predictive Modeling
With the data analyzed, predictive modeling can forecast future churn. This involves:
- Model Training: Using historical data to train machine learning models. The model learns to associate certain behaviors and patterns with churn.
- Model Validation: Testing the model with a subset of data to ensure its accuracy and reliability.
- Scoring: Assigning churn risk scores to each customer based on the model’s predictions. High scores indicate a higher likelihood of churn.
5. Intervention Strategies
The final element is to develop and implement strategies to mitigate churn. Based on the risk scores and identified predictors, businesses can:
- Personalized Outreach: Engage at-risk customers with personalized communication and offers.
- Incentives: Provide discounts, extended trials, or loyalty rewards to retain customers.
- Enhanced Support: Offer dedicated support to resolve issues and improve customer satisfaction.
- Re-engagement Campaigns: Use targeted marketing efforts to re-engage customers showing signs of disengagement.
By integrating these elements, businesses can create a comprehensive churn prediction model that not only forecasts customer attrition but also provides actionable insights to enhance customer retention. This proactive approach ensures that efforts are focused on retaining valuable customers, ultimately contributing to sustained business growth and success.
Churn Prediction Modeling with Lead Scoring and Salespanel
Salespanel tracks customer behavior for B2B businesses and can help web-hosted products and services like SaaS companies analyze behavior, predict churn, and implement effective retention strategies.
Analyzing Churned Customer Behavior
The first step in using Salespanel for churn prediction involves analyzing the behavior of customers who have already churned. By examining their usage patterns, engagement levels, support interactions, and feedback, businesses can identify common traits and warning signs that precede churn. This historical analysis is essential for setting up predictive rules and scoring systems.
Lead Scoring to Predict Churn
Lead scoring assigns numerical values to various customer behaviors and attributes, helping prioritize retention efforts. With Salespanel, you can set up a lead scoring system that incorporates identified churn indicators. For example:
- Decreased Engagement: Assign higher scores to customers who log in less frequently or show reduced usage of key features.
- Negative Feedback: Track and score high volumes of complaints or negative feedback.
- Support Requests: Score based on the number and nature of support tickets, particularly if they are related to recurring issues.
- Non-Renewal Signals: Higher scores for customers delaying renewals or showing interest in competitors.
These scores help Salespanel alert you when a customer’s risk of churn reaches a critical level, allowing for timely and targeted actions.
Automated Interventions and Integrations
Salespanel’s integration capabilities enable automated retention strategies for at-risk customers. Some effective methods include:
- Email Drip Campaigns: Send personalized email sequences to re-engage customers, offering valuable content or special discounts.
- Chat Popups: Implement automated chat popups to offer immediate assistance or special deals when at-risk customers visit your site.
- Discount Offers: Provide automatic discounts or special deals to disengaged customers as incentives to stay.
- Consultation Offers: Offer free consultations or personalized support sessions to address any issues or concerns driving dissatisfaction.
Churn prediction is an ongoing process. Regularly update your lead scoring rules and automated interventions based on new data and insights to ensure your churn prediction model remains accurate and effective. By combining churn prediction modeling with lead scoring and the automation capabilities of Salespanel, B2B businesses can significantly enhance their customer retention efforts. This proactive approach not only reduces churn rates but also fosters stronger customer relationships and drives long-term growth.
Role of Machine Learning and AI in Churn Prediction
The rapid growth and adoption of artificial intelligence (AI) and machine learning (ML) are transforming customer churn prediction. These technologies provide more dynamic and accurate predictions compared to traditional statistical methods, enabling businesses to foresee customer behavior with greater confidence.
Through supervised learning, ML algorithms can be trained on historical customer data to recognize patterns and early warning signs of dissatisfaction or disengagement. By processing vast amounts of data, they can uncover complex relationships that traditional methods might miss, allowing for highly accurate predictions of which customers are at risk of churning.
AI enhances this process by continuously learning and adapting to new data, allowing for real-time insights as customer interactions occur. This continuous learning ensures that predictive models remain relevant and accurate even as customer preferences and behaviors evolve. With up-to-date predictions, businesses can implement proactive retention strategies tailored to individual customers.
AI and ML enable personalized marketing efforts that address specific pain points and preferences, such as automatically sending targeted offers or support messages to disengaged customers. These personalized interventions enhance customer experience, making customers feel valued and understood, thereby reducing the likelihood of churn.
Moreover, AI and ML help improve overall customer experiences by analyzing customer data to identify common issues and areas for improvement in products or services. Businesses can use these insights to make informed decisions about product enhancements and customer service improvements, directly impacting customer satisfaction. Addressing the root causes of churn creates a more positive and engaging experience for customers, fostering long-term loyalty.
Additionally, these technologies offer scalability and efficiency, capable of handling large volumes of data quickly. This scalability ensures that businesses of all sizes can effectively monitor and reduce churn, even as they grow. In conclusion, AI and ML integration in churn prediction significantly advances customer retention strategies, providing enhanced accuracy, real-time insights, proactive interventions, and improved customer experiences, thus helping businesses maintain strong customer relationships and achieve sustainable growth.
Final Thoughts
Effectively predicting and managing customer churn is crucial for maintaining a stable and profitable business. By leveraging advanced data analytics, machine learning, and AI, businesses can accurately forecast which customers are at risk of leaving. Tools like Salespanel further enhance these capabilities by providing real-time insights and enabling automated, personalized retention strategies. Understanding and addressing the root causes of churn not only helps in retaining valuable customers but also improves overall customer satisfaction and loyalty. By implementing a robust churn prediction system, businesses can ensure sustained growth, foster long-term relationships, and maintain a competitive edge in the market.
Sell more, understand your customers’ journey for free!
Sales and Marketing teams spend millions of dollars to bring visitors to your website. But do you track your customer’s journey? Do you know who buys and why?
Around 8% of your website traffic will sign up on your lead forms. What happens to the other 92% of your traffic? Can you identify your visiting accounts? Can you engage and retarget your qualified visitors even if they are not identified?